
Motivation
Automatic prediction of the list of species most likely to be observed at a given location is useful for many scenarios related to biodiversity management and conservation. First, it could improve species identification tools (whether automatic, semi-automatic or based on traditional field guides) by reducing the list of candidate species observable at a given site. More generally, this could facilitate biodiversity inventories through the development of location-based recommendation services (e.g. on mobile phones), encourage the involvement of citizen scientist observers, and accelerate the annotation and validation of species observations to produce large, high-quality data sets. Last but not least, this could be used for educational purposes through biodiversity discovery applications with features such as contextualized educational pathways.
Data collection
The challenge will rely on a collection of millions of occurrences of plants and animals in the US and France (primarily from GBIF , iNaturalist , Pl@ntNet and a few expert collections). In addition to geo-coordinates and species name, each occurrence will be matched with a set of geographic images characterizing the local landscape and environment around the occurrence. In more detail, this will include: (i) high resolution (about 1 meter per pixel) remotely sensed imagery (from NAIP for the US and from IGN for France, (ii) bio-climatic rasters from WorldClim (1 km resolution) and (iii), land cover rasters (from NLCD for the US (30m resolution) and from Cesbio for France (10m resolution).
Task description
The detailed description of the challenge is provided on the AICrowd page of the challenge: GeoLifeCLEF 2020 .
In a nutshell, the occurrence dataset is split in a training set with known species name labels and a test set used for the evaluation. For each occurrence in the test set (paired with the corresponding satellite image and environmental co-variates), the goal of the task will is to return a candidate set of species with associated confidence scores. The evaluation metric will be an adaptive top-K accuracy.
How to participate ?
See registrations instructions here. Fast link to the GeoLifeCLEF challenge on AICrowd: GeoLifeCLEF 2020
Reward
The winner of each of the four LifeCLEF 2020 challenges will be offered a cloud credit grants of 5k USD as part of Microsoft's AI for earth program.

Results
The overview paper presenting the results of the challenge is available here (ceur-ws proceeedings)
Two participants submitted a total of 8 runs but only 3 runs were finally considered as valid:

The method achieving the best results (LIRMM Submission 3) was based solely on a convolutional neural network (CNN) trained on the high-resolution covariates (RGB-IR imagery, land cover, and altitude). It did not make use of any bioclimatic or soil variables, which are often considered to be the most informative in the ecological literature. On the contrary, LIRMM Submission 1 was a machine learning method classically used for species distribution models (Random Forest) trained solely on the climatic and soil variables. Submission 3 of Stanford was a baseline method that always predicted the list of the most frequent species in the training set.
Credits
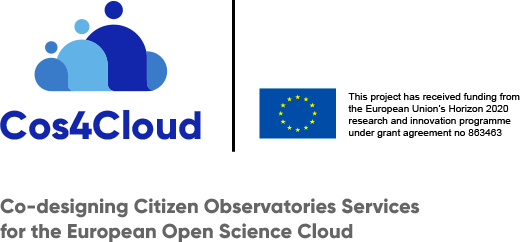