Schedule
- December 2024: Registration opens for all LifeCLEF challenges Registration is free of charge
- 1 February 2025: Competition Start
- 5 May 2025: Competition Deadline
- 19 May 2025: Deadline for submission of working note papers by participants [CEUR-WS proceedings]
- 23 June 2025: Notification of acceptance of working note papers [CEUR-WS proceedings]
- 30 June 2025: Camera-ready deadline for working note papers.
- 9-12 Sept 2025: CLEF 2025 Madrid - Spain
All deadlines are at 11:59 PM CET on a corresponding day unless otherwise noted. The competition organizers reserve the right to update the contest timeline if they deem it necessary.
Motivation
Automatic recognition of fungi species aids mycologists, citizen scientists, and nature enthusiasts in identifying species in the wild while supporting the collection of valuable biodiversity data. To be effective on a large scale, such as in popular citizen science projects, it needs to efficiently predict species with limited resources and handle many classes, some of which have just a few recorded observations. Additionally, rare species are often excluded from training, making it difficult for AI-powered tools to recognize them. Based on our measurements, we recognized that about 20\% of all verified observations (20,000) involve rare or under-recorded species, highlighting the need to accurately identify these species.
Task Description
TBD
Participation requirements
TBD
Publication Track
All registered participants are encouraged to submit a working-note paper to peer-reviewed LifeCLEF proceedings (CEUR-WS) after the competition ends.
This paper must provide sufficient information to reproduce the final submitted runs.
Only participants who submitted a working-note paper will be part of the officially published ranking used for scientific communication.
The results of the campaign appear in the working notes proceedings published by CEUR Workshop Proceedings (CEUR-WS.org).
Selected contributions among the participants will be invited for publication in the Springer Lecture Notes in Computer Science (LNCS) the following year.
Data
TBD
Evaluation process
TBD
Organizers
Machine Learning
- Lukas Picek, INRIA--Montpellier, France & Dept. of Cybernetics, FAV, University of West Bohemia, Czechia, lukaspicek@gmail.com
- Klára Janoušková, The Center for Machine Perception Dept. of Cybernetics, FEE, Czech Technical University, Czechia, klara.janou@gmail.com
- Jiri Matas, The Center for Machine Perception Dept. of Cybernetics, FEE, Czech Technical University, Czechia, matas@cmp.felk.cvut.cz
Mycology
- Jacob Heilmann-Clausen, Center for Macroecology, Evolution and Climate; University of Copenhagen, Denmark, jheilmann-clausen@sund.ku.dk
Credits
Acknowledgement
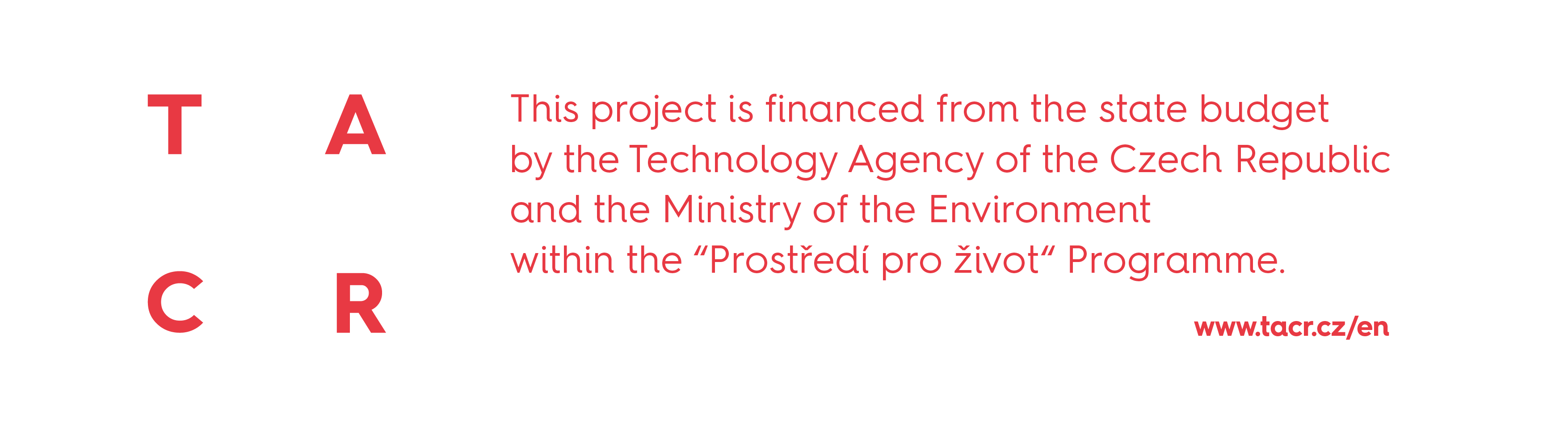
