
News |
A direct link to the overview of the task:
LifeCLEF Plant Identification Task 2014, CLEF 2014 working notes, Goëau H., Joly A., Bonnet P., Selmi S., Molino J-F., Barthélémy D. and Boujemaa N., LifeCLEF 2014 working notes, Sheffield, United Kingdom. |
10.02.2015 The public packages containing all the data of the LifeCLEF 2014 plant retrieval task are now available (including the ground truth, an executable in order to compute new scores, the working notes and some additional informations). The entire data is under Creative Common license. You can download it using the following urls:
https://lab.plantnet.org/LifeCLEF/PlantCLEF2014/TrainPackages/PlantCLEF2...
https://lab.plantnet.org/LifeCLEF/PlantCLEF2014/TestPackages/PlantCLEF20...
https://lab.plantnet.org/LifeCLEF/PlantCLEF2014/TestPackages/PlantCLEF20...
|
Context
If agricultural development is to be successful and biodiversity is to be conserved, then accurate knowledge of the identity, geographic distribution and uses of plants is essential. Unfortunately, such basic information is often only partially available for professional stakeholders, teachers, scientists and citizens, and often incomplete for ecosystems that possess the highest plant diversity. So that simply identifying plant species is usually a very difficult task, even for professionals (such as farmers or wood exploiters) or for the botanists themselves. Using image retrieval technologies is nowadays considered by botanists as a promising direction in reducing this taxonomic gap. Evaluating recent advances of the IR community on this challenging task might therefore have a strong impact. The organization of this task is funded by the French project Pl@ntNet (INRIA, CIRAD, Telabotanica) and the French CNRS program MASTODONS.
Task Overview
Following the success of the three previous ImageCLEF 2011-13 plant identification tasks, we are glad to organize this year a new challenge dedicated to botanical data. The task will be focused on tree, herbs and ferns species identification based on different types of images. Main novelties compared to the last years are the following (see bellow for more explanations):
- an explicit multi-image query scenario
- user ratings on image quality
- a new type of view called "Branch" additionally to the 6 others views: Scan (scan or scan-like pictures of leaf), photos of Flower, Fruit, Stem, Leaf and Entire views
- and basically more species: the number of species will be this year about 500, which is an important step towards covering the entire flora of a given region.
Multi-image query
The motivation of the task is to fit better with a real scenario where one user tries to identify a plant by observing its different organs, such as it has been demonstrated in [MAED2012]. Indeed, botanists usually observe simultaneously several organs like the leaves and the fruits or the flowers in order to disambiguate species which could be confused if only one organ were observed. Moreover, if only one organ is observed, such as the bark of a deciduous plant during winter where nothing else is observable, then the observation of this organ with several photos related to different point of views could be more informative than only one point of view.
Thus, contrary to the previous years, the species identification task won't be image-centered but OBSERVATION-centered. The aim of the task will be to produce a list of relevant species for each observation of a plant of the test dataset, i.e. one or a set of several pictures related to a same event: one same person photographing several detailed views on various organs the same day with the same device with the same lightening conditions observing one same plant.

One observation of one individual-plant observed the same day by a same author involving several pictures with the same Observation ID. If this observation is in the test dataset, participants of the task will have to produce a list of relevant species according to the set of the 6 pictures.
User ratings on image quality
The training and test data will be composed of images collected through a citizen sciences initiative that was initiated 4 years ago in collaboration with Tela Botanica (social network of amateur and expert botanists). This makes the task closer to the conditions of a real-world application: (i) images of the same species are coming from distinct plants living in distinct areas (ii) pictures are taken by different users that might not used the same protocol to acquire the images (iii) pictures are taken at different periods in the year. Additional information will include contextual meta-data (author, date, locality name) and some EXIF data.
An important novelty this year is the vote annotation for most of the pictures. These annotations are collectively produced by the Tela Botanica members through a collaborative web tool called pictoflora released one year ago now.
The following table gives some examples of pictures with the average of the user ratings on image quality, and illustrates at the same time the different types of views (the "LeafScan" views, the photographs of "Leaf", "Flower", "Fruit", "Stem", "Entire", and the new view called "Branch"). More the value is close to 5, more the organ is well photographed, typically a close-up photo where the organ covers a wide surface of the picture, sharp while the background is optically blurred thanks to a short deep-of-field, and thus with a clear and useful visual content for helping the plant identification. At the opposite, more the value is close to 1, less the picture is helpful for identifying the species for various reasons: the picture is globally blurred or the organ is out of focus, the organ is to small or/and the background is predominant with a sharp visual content like grass or leafage of other plants, the organ is too damaged like old fruit or dry leaves, some external object like a ruler, a pen or a coin for giving some information on size, etc.
Participants will be free to exploit or not all the pictures of the training dataset according to these user rating informations.
Stars |
 |
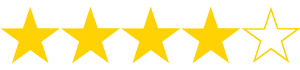 |
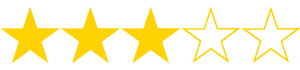 |
 |
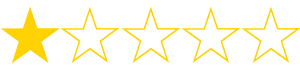 |
Branch Cercis silliquastrum L. |
 |
 |
 |
 |
 |
Entire Quercus ilex L. |
 |
 |
 |
 |
 |
Leaf (photo) Pittosporum tobira L. |
 |
 |
 |
 |
 |
Leaf (scan & scan-like) Hedera helix L. |
 |
 |
 |
 |
 |
Flower Papaver rhoeas L. |
 |
 |
 |
 |
 |
Fruit Crataegus monogyna L. |
 |
 |
 |
 |
 |
Stem Betula pendula L. |
 |
 |
 |
 |
 |
All the dataset is built and produced collaboratively by the Tela Botanica members: the quality votes, but also the view tags on each pictures, and the taxonomical names associated to the observations through a second online tool called Identiplante. It means that the annotations, the rates and the species names are revised and slightly improved days by days, but some errors can occur in the dataset.
Dataset
The task will be based on the Pl@ntView dataset which focuses on 500 herb, tree and fern species centered on France (some plants observations are from neighboring countries). It contains more than 60000 pictures belonging each to one of the 7 types of view reported into the meta-data, in a xml file (one per image) with explicit tags.
To sum up each image is associated with the following meta-data:
- ObservationId: the plant observation ID from which several pictures can be associated
- FileName
- MediaId
- View Content: Branch, Entire, Flower, Fruit, Leaf, LeafScan, Stem
- ClassId: the class number ID that must be used as ground-truth. It is a numerical taxonomical number used by Tela Botanica
- Species the species names (containing 3 parts: the Genus name, the Species name, the author(s) who discovered or revised the name of the species)
- Genus: the name of the Genus, one level above the Species in the taxonomical hierarchy used by Tela Botanica
- Family: the name of the Family, two levels above the Species in the taxonomical hierarchy used by Tela Botanica
- Date: (if available) the date when the plant was observed,
- Vote: the (round up) average of the user ratings on image quality
- Locality: (if available) locality name, most of the time a town
- Latitude & Longitude: (if available) the GPS coordinates of the observation in the EXIF metadata, or, if no GPS information were found in the EXIF, the GPS coordinates of the locality where the plant was observed (only for the towns of metropolitan France)
- Author: name of the author of the picture,
And if the image was included in previous plant task:
- Year: ImageCLEF2011, ImageCLEF2012, ImageCLEF2013, PlantCLEF2014 when the image was integrated in the benchmark
- IndividualPlantId2013: the plant observation ID used last year during the ImageCLEF2013 plant task,
- ImageID2013: the image id.jpg used in 2013.
We provide here a set of 2 files related to one image: the picture and its associated xml file:
- 6.jpg
- 6.xml
Partial meta-data information can be found in the image's EXIF, and might include:
- the camera or the scanner model,
- the image resolutions and the dimensions,
- for photos, the optical parameters, the white balance, the light measures…
All data are published under a creative commons license.
Please notice that, to allow a fair evaluation, it will be strictly forbidden to use more external online resources as training data to enrich the provided one.
Task description
Goal
The task will be evaluated as a plant species retrieval task based on multi-image plant observations queries. The goal is to retrieve the correct plant species among the top results of a ranked list of species returned by the evaluated system. Contrary to previous plant identification benchmarks, queries are not defined as single images but as plant observations, meaning a set of 1 to 5 images depicting the same individual plant observed by the same person the same day. Each image of a query observation is associated with a single view type (entire plant, branch, leaf, fruit, flower, stem or leaf scan) and with contextual meta-data (data, location, author). Each participating group is allowed to submit up to 4 runs built from different methods. Semi-supervised and interactive approaches, particularly for segmenting leaves from the background, are allowed but will be compared independently from fully automatic methods. Any human assistance in the processing of the test queries has therefore to be signaled in the submitted runs (see next section on how to do that).
Training and test data
A part of PlantCLEF dataset will be provided as training data whereas the remaining part will be used later as test data. Training AND test pictures used during ImageCLEF 2013 Plant Identification Task are mostly part from the dataset and they will be systematically included in the training set. Test observation will be chosen by randomly sampling 1/3 of the observations of each species.
- The training data finally results in 47815 images (1987 of "Branch", 6356 photographs of "Entire", 13164 of Flower", 3753 "Fruit", 7754 of "Leaf", 3466 "Stem" and 11335 scans and scan-like pictures of leaf) with complete xml files associated to them. Download link of training data will be sent to participants on 15/01/2014.
- The test data results in 8163 plant-observation-queries. These queries are based on 13146 images (731 of "Branch", 2983 photographs of "Entire", 4559 of Flower", 1184 "Fruit", 2058 of "Leaf", 935 "Stem" and 696 scans and scan-like pictures of leaf) with purged xml files, i.e without the taxon name (the ground truth), the vernacular name (common name of the plant) and the image quality ratings (that would not be available at query stage in a real-world mobile application).
The queries are expressed in the ObservationId field in the purged xml, but an explicitly list of queries can be found here: http://www.imageclef.org/system/files/QueriesObservationId_0.txt
The link between the queries and the images are expressed with the ObservationId and FileName fields in each purged xml files, but an explicitly map between the test images and the queries can be found here:
http://www.imageclef.org/system/files/TestImageToObservationIdQuery.txt
Participants are allowed to train distinct classifiers, use different training subsets or use distinct methods for each type of view.
Run format
The run file must be named as "teamname_runX.run" where X is the identifier of the run (i.e. 1, 2, 3 or 4). The run file has to contain as much lines as the total number of predictions, with at least one prediction per test observation plant and a maximum of 500 predictions per test image (500 being the total number of species). Each prediction item (i.e. each line of the run file) has to respect the following format:
<ObservationId;ClassId;rank;score>
The ClassId is the main taxonomical number in an official french taxonomical referential used by Tela Botanica and helping botanists to deal with synonymy. <rank> is the ranking of a given species for a given test ObservationId. <Score> is a confidence score of a prediction item (the lower the score the lower the confidence). Here is a short fake run example respecting this format on only 8 test observations:
myteam_run3.txt
The order of the prediction items (i.e. the lines of the run file) has no influence on the evaluation metric, so that contrary to our example prediction items might be sorted in any way. On the other side, the <rank> field is the most important one since it will be used as the main key to sort species and compute the final metric.
For each submitted run, please give in the submission system a description of the run. A combobox will specify wether the run was performed fully automatically or with a human assistance in the processing of the queries. Then, a textarea should contain a short description of the used method, particularly for helping differentiating the different runs submitted by the same group, for instance:
selecting only pictures with a vote >1 in the training dataset, and for each image of the test observation plant: matching-based method using SIFT features, RANSAC algorithm and K-NN classifier with K=20 in order to produce a probability distribution. Then bayesian rule for combining the probabilities for producing a species list following a decreasing order of final probabilities
Optionally, you can add one or several bibtex reference(s) to publication(s) describing the method more in details.
For each submitted run, it will be asked (optional but highly recommended) to produce a second run file detailing species prediction at image level like previous years, before the combination of multiple-image of the observation query. This complementary run file must be named as "teamname_runX_image.run" where X is the identifier of the run (i.e. 1,2 or 3). The run file has to contain as much lines as the total number of predictions, with at least one prediction per test image and a maximum of 500 predictions per test image (500 being the total number of species). Each prediction item (i.e. each line of the file) has to respect the following format :
<test_image_name.jpg;ClassId;rank;score>
Here is a fake run example respecting this format:
myteam_run3_image.txt
This optional file will be very useful in order to analysis how much the combination of pictures from a same plant contribute to the final contribution comparing a single image species prediction. Moreover, it will enable to produce detailed scores by type of view like the three previous years.
Metrics
The primary metric used to evaluate the submitted runs will be a score related to the rank of the correct species in the list of retrieved species. Each plant observation test will be attributed with a score between 0 and 1: of 1 if the 1st returned species is correct and will decrease quickly while the rank of the correct species increases. An average score will then be computed on all test individual plant. A simple mean on all plant observation test would however introduce some bias. Indeed, we remind that the PlantCLEF dataset was built in a collaborative manner. So that few contributors might have provided much more observations and pictures than many other contributors who provided few. Since we want to evaluate the ability of a system to provide correct answers to all users, we rather measure the mean of the average classification rate per author. Finally, our primary metric is defined as the following average classification score S:
U : number of users (who have at least one image in the test data)
Pu : number of individual plants observed by the u-th user
Su,p : score between 1 and 0 equals to the inverse of the rank of the correct species (for the p-th plant observed by the u-th user)
A secondary metric will be used to evaluate the complementary submitted runs providing species determinations at the image level. Each test image will be attributed with a score between 0 and 1 : of 1 if the 1st returned species is correct and will decrease quickly while the rank of the correct species increases. An average score will then be computed on all test images. Following the same motivations express above, a simple mean on all test images would however introduce some bias. Some authors sometimes provided many pictures of the same individual plant (to enrich training data with less efforts). Since we want to evaluate the ability of a system to provide the correct answer based on a single plant observation, we also have to average the classification rate on each individual plant. Finally, our secondary metric is defined as the following average classification score S:
U : number of users (who have at least one image in the test data)
Pu : number of individual plants observed by the u-th user
Nu,p : number of pictures taken from the p-th plant observed by the u-th user
Su,p,n : score between 1 and 0 equals to the inverse of the rank of the correct species (for the n-th picture taken from the p-th plant observed by the u-th user)
How to register for the task
LifeCLEF will use the ImageCLEF registration interface. Here you can choose a user name and a password. This registration interface is for example used for the submission of runs. If you already have a login from the former ImageCLEF benchmarks you can migrate it to LifeCLEF 2014 here
Schedule
- 01.12.2013: Registration opens (register here)
- 15.01.2014: training data release
- 19.03.2014: test data release
08.05.201415.05.2014: deadline for submission of runs
15.05.201422.05.2014:release of results
- 07.06.2014: deadline for submission of working notes
- 15-18.09.2014: CLEF 2014 Conference (Sheffield, UK)
Frequently asked questions
In the "test" dataset there are associated xml files where "Content" attributes are indicated. Are we allowed to use this information during the prediction task or would it be considered as a manual intervention on the process.
Yes, you are allowed to use this information during the prediction (like in the two previous years). We consider that species identification is a very challenging task and we don't want to add more difficulties with an organ/view prediction step.
Results
A total of 10 participating groups submitted 27 runs focusing on plant observations. 6 teams submitted 14 complementary runs on images. Thanks to all of you for your efforts and your constructive feedbacks regarding the organization.
Main task
The following graphic and table show the scores obtained on the main task on multi-image plant observation queries.
<
Run name |
Run filename |
Score |
IBM AU Run 4 |
1400024261057__ibmau_run4 |
0,471 |
IBM AU Run 3 |
1399956011853__ibmau_run3 |
0,459 |
IBM AU Run 2 |
1399341122320__ibmau_run2 |
0,454 |
PlantNet Run 2 |
1400190690390__PlantNet_run2 |
0,289 |
PlantNet Run 3 |
1400191097248__PlantNet_run3 |
0,289 |
PlantNet Run 4 |
1400192000624__PlantNet_run4 |
0,282 |
PlantNet Run 1 |
1400192943635__PlantNet_run1 |
0,278 |
IBM AU Run 1 |
1399340349340__ibmau_run1 |
0,271 |
BME TMIT Run 1 |
1400149655446__bme-tmit_run1 |
0,255 |
BME TMIT Run 2 |
1400156045211__bme-tmit_run2 |
0,255 |
BME TMIT Run 3 |
1400156500287__bme-tmit_run3 |
0,255 |
QUT Run 1 |
1400132196272__QUT_run1 |
0,249 |
FINKI Run 1 |
1400136735418__run_maestra_mixed |
0,205 |
FINKI Run 3 |
1400142101128__run_maestra_prob |
0,204 |
FINKI Run 2 |
1400141893031__run_maestra_min_rank |
0,166 |
Sabanci Okan Run 1 |
1400183415332__Sabanci-Okan_run1 |
0,127 |
Sabanci Okan Run 2 |
1400186247207__Sabanci-Okan_run2 |
0,127 |
I3S Run 1 |
1400054588907__mind_run1 |
0,091 |
I3S Run 2 |
1400146846184__mind_run2 |
0,089 |
SZTE Run 1 |
1399379800927__run1 |
0,088 |
SZTE Run 3 |
1399381417968__run3 |
0,086 |
SZTE Run 2 |
1399380824176__run2 |
0,085 |
SZTE Run 4 |
1399381815308__run4 |
0,085 |
Miracl Run 1 |
miracl_run1 |
0,063 |
Miracl Run 2 |
miracl_run2 |
0,051 |
Miracl Run 3 |
miracl_run3 |
0,047 |
IV Processing Run 1 |
IVprocessing_run1 |
0,043 |
Complementary results on images
The following graphic and table and below present the scores obtained on the complementary run files focusing on images. Thanks to the participants who produced these not mandatory run files. In order to evaluate the benefit of the combination of the test images from the same observation, the graphic compares the pairs of run files on images and on observations assumed to have been produced with the same method.
(Click on the graphic to enlarge it.)
Run name |
Run filename |
Score Image |
Score Observation |
IBM AU Run 4 |
1400024432284__ibmau_run4_image |
0,456 |
0,471
|
IBM AU Run 3 |
1399956119246__ibmau_run3_image |
0,446 |
0,459
|
IBM AU Run 2 |
1399943893376__ibmau_run2_image |
0,438 |
0,454
|
PlantNet Run 2 |
1400190941511__PlantNet_run2_image |
0,28 |
0,289
|
PlantNet Run 4 |
1400191994221__PlantNet_run4_image |
0,276 |
0,282
|
PlantNet Run 1 |
1400191748018__PlantNet_run1_image |
0,271 |
0,278
|
IBM AU Run 1 |
1399943759499__ibmau_run1_image |
0,263 |
0,271
|
FINKI Run 1 |
1400141784337__run_maestra_per_image_mixed |
0,205 |
0,205
|
FINKI Run 3 |
1400142192439__run_maestra_per_image_prob |
0,204 |
0,204
|
FINKI Run 2 |
1400141974924__run_maestra_per_image_min_rank |
0,166 |
0,166
|
Sabanci Okan Run1 |
1400190140405__Sabanci-Okan_run1_image |
0,123 |
0,127
|
Sabanci Okan Run2 |
1400190220805__Sabanci-Okan_run2_image |
0,123 |
0,127
|
BME TMIT Run 1 |
1400157686528__bme-tmit_run1_image |
0,086 |
0,255
|
I3S Run 1 |
1400056844108__mind_run1_image |
0,043 |
0,091
|
Complementary results on images detailed by organs
The following table and graphics below show the detailed scores obtained for each type of plant organs. Remember that we use a specific metric weighted by authors and plants, and not by sub-categories, explaining why the score on images not detailed is not the mean of the 7 scores of these sub-categories.
(Click on the graphics to enlarge them.)
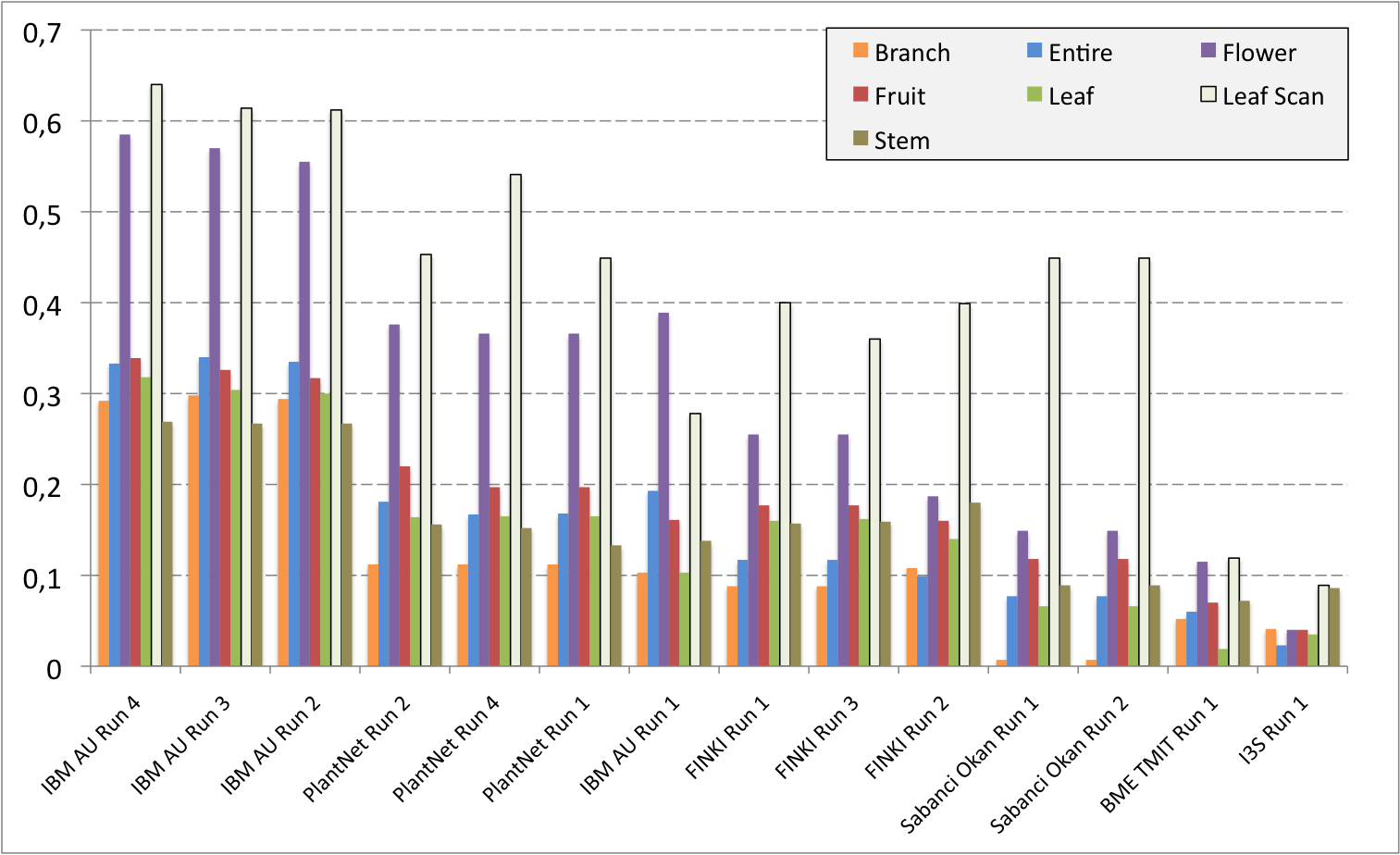
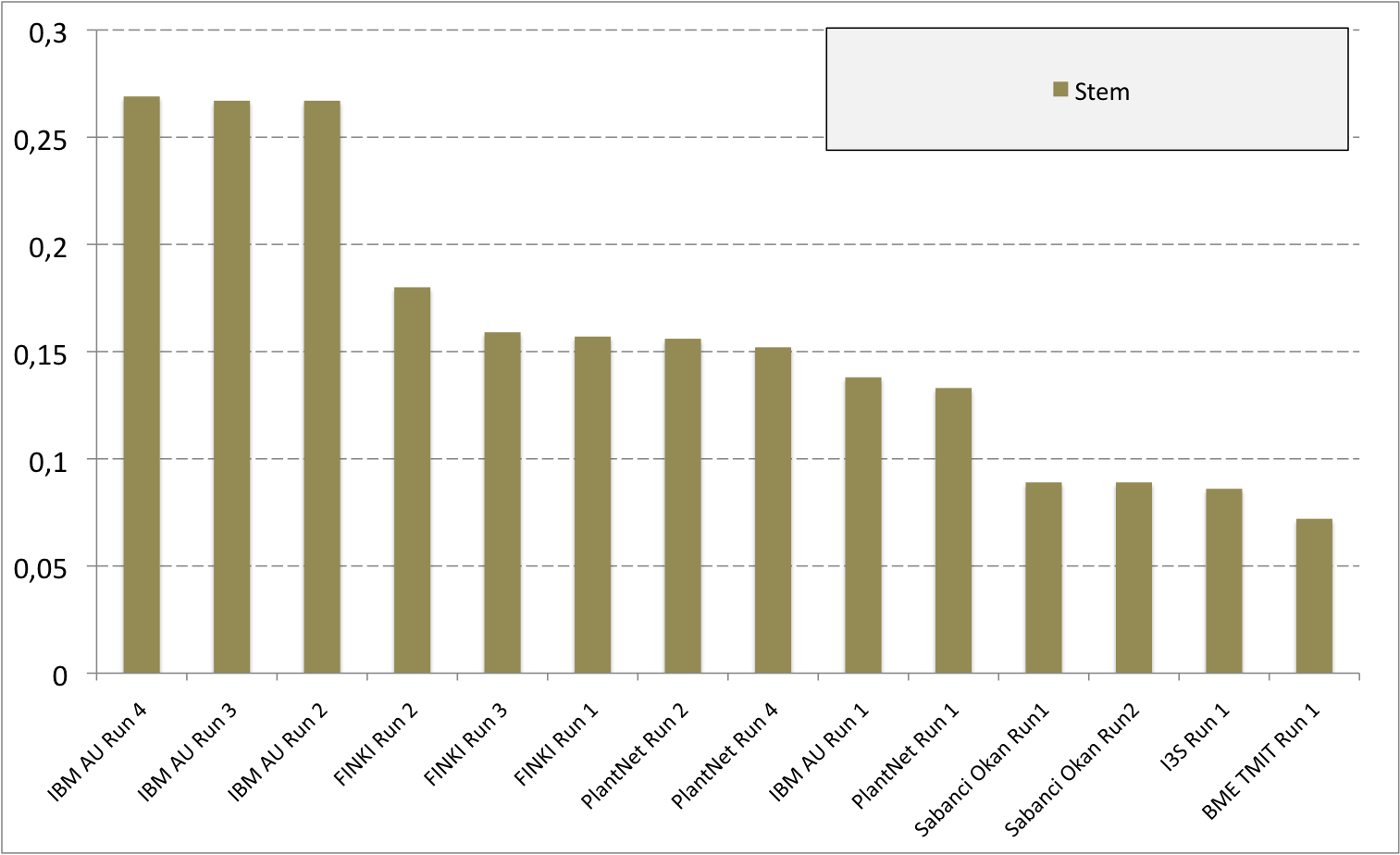
Run name |
Run filename |
Run name |
Branch |
Entire |
Flower |
Fruit |
Leaf |
Leaf Scan |
Stem |
IBM AU Run 4 |
1400024432284__ibmau_run4_image |
IBM AU Run 4 |
0,292 |
0,333 |
0,585 |
0,339 |
0,318 |
0,64 |
0,269
|
IBM AU Run 3 |
1399956119246__ibmau_run3_image |
IBM AU Run 3 |
0,298 |
0,34 |
0,57 |
0,326 |
0,304 |
0,614 |
0,267
|
IBM AU Run 2 |
1399943893376__ibmau_run2_image |
IBM AU Run 2 |
0,294 |
0,335 |
0,555 |
0,317 |
0,3 |
0,612 |
0,267
|
PlantNet Run 4 |
1400191994221__PlantNet_run4_image |
PlantNet Run 4 |
0,112 |
0,167 |
0,366 |
0,197 |
0,165 |
0,541 |
0,152
|
PlantNet Run 2 |
1400190941511__PlantNet_run2_image |
PlantNet Run 2 |
0,112 |
0,181 |
0,376 |
0,22 |
0,164 |
0,453 |
0,156
|
PlantNet Run 1 |
1400191748018__PlantNet_run1_image |
PlantNet Run 1 |
0,112 |
0,168 |
0,366 |
0,197 |
0,165 |
0,449 |
0,133
|
Sabanci Okan Run1 |
1400190140405__Sabanci-Okan_run1_image |
Sabanci Okan Run1 |
0,007 |
0,077 |
0,149 |
0,118 |
0,066 |
0,449 |
0,089
|
Sabanci Okan Run2 |
1400190220805__Sabanci-Okan_run2_image |
Sabanci Okan Run2 |
0,007 |
0,077 |
0,149 |
0,118 |
0,066 |
0,449 |
0,089
|
FINKI Run 1 |
1400141784337__run_maestra_per_image_mixed |
FINKI Run 1 |
0,088 |
0,117 |
0,255 |
0,177 |
0,16 |
0,4 |
0,157
|
FINKI Run 2 |
1400141974924__run_maestra_per_image_min_rank |
FINKI Run 2 |
0,108 |
0,099 |
0,187 |
0,16 |
0,14 |
0,399 |
0,18
|
FINKI Run 3 |
1400142192439__run_maestra_per_image_prob |
FINKI Run 3 |
0,088 |
0,117 |
0,255 |
0,177 |
0,162 |
0,36 |
0,159
|
IBM AU Run 1 |
1399943759499__ibmau_run1_image |
IBM AU Run 1 |
0,103 |
0,193 |
0,389 |
0,161 |
0,103 |
0,278 |
0,138
|
BME TMIT Run 1 |
1400157686528__bme-tmit_run1_image |
BME TMIT Run 1 |
0,052 |
0,06 |
0,115 |
0,07 |
0,019 |
0,119 |
0,072
|
I3S Run 1 |
1400056844108__mind_run1_image |
I3S Run 1 |
0,041 |
0,023 |
0,04 |
0,04 |
0,035 |
0,089 |
0,086
|
____________________________________________________________________________________________________________________
Contacts
Hervé Goeau (INRIA-ZENITH, INRIA-IMEDIA): herve(replace-that-by-a-dot)goeau(replace-that-by-an-arrobe)inria.fr
Alexis Joly (INRIA-ZENITH): alexis(replace-that-by-a-dot)joly(replace-that-by-an-arrobe)inria.fr
Pierre Bonnet (AMAP): pierre(replace-that-by-a-dot)bonnet(replace-that-by-an-arrobe)cirad.fr