News
- 04.08.2012 Results for the image-based and case-base retrieval task are released.
- 13.07.2012 Results for the modality classification task are released.
- 17.04.2012 Training data for the modality classification task is released.
- 20.03.2012 Data for the medical task is released.
- 15.2.2012 Registration of ImageCLEF 2012 is open.
- 1.12.2011 ImageCLEF is accepted as CLEF 2012 lab.
Schedule:
- 15.2.2012: registration opens for all ImageCLEF tasks
- 15.3.2012: data release
- 17.4.2012: training data release for the modality classification task
- 10.5.2012: topic release for the retrieval tasks
- 15.6.2012: submission of runs
- 15.7.2012: release of results
- 17.8.2012: submission of working notes papers
- 17.09.2012-20.09.2012: CLEF 2012 Conference, Rome, Italy
Citations
- When referring to ImageCLEFmed 2012 task general goals, general results, etc. please cite the following publication:
- Henning Müller, Alba García Seco de Herrera, Jayashree Kalpathy-Cramer, Dina Demner-Fushman, Sameer Antani and Ivan Eggel, Overview of the ImageCLEF 2012 medical image retrieval and classification tasks, in: CLEF 2012 working notes, 2012
-
BibText:
@InProceedings{MGK2012,
Title = {Overview of the {ImageCLEF} 2012 Medical Image Retrieval and Classification Tasks},
Author = {M\"uller, Henning and Garc\'ia Seco de Herrera, Alba and Kalpathy--Cramer, Jayashree and Demner Fushman, Dina and Antani, Sameer and Eggel, Ivan},
Booktitle = {Working Notes of {CLEF} 2012 (Cross Language Evaluation Forum)},
Year = {2012},
Month = {September},
Location = {Rome, Italy}
}
- When referring to ImageCLEFmed task in general, please cite the following publication:
-
Jayashree Kalpathy-Cramer, Alba García Seco de Herrera, Dina Demner-Fushman, Sameer Antani, Steven Bedrick and Henning Müller, Evaluating Performance of Biomedical Image Retrieval Systems –an Overview of the Medical Image Retrieval task at ImageCLEF 2004-2014 (2014), in: Computerized Medical Imaging and Graphics
-
BibText:
@Article{KGD2014,
Title = {Evaluating Performance of Biomedical Image Retrieval Systems-- an Overview of the Medical Image Retrieval task at {ImageCLEF} 2004--2014},
Author = {Kalpathy--Cramer, Jayashree and Garc\'ia Seco de Herrera, Alba and Demner--Fushman, Dina and Antani, Sameer and Bedrick, Steven and M\"uller, Henning},
Journal = {Computerized Medical Imaging and Graphics},
Year = {2014}
}
Tasks overview
The medical retrieval task of ImageCLEF 2012 uses a subset of PubMed Central containing 305,000 images.
This task is a use case of the Promise network of excellence and supported by the project.
There will be three types of tasks in 2012:
- Modality Classification:
Previous studies have shown that imaging modality is an important aspect of the image for medical retrieval. In user-studies, clinicians have indicated that modality is one of the most important filters that they would like to be able to limit their search by. Many image retrieval websites (Goldminer, Yottalook) allow users to limit the search results to a particular modality. However, this modality is typically extracted from the caption and is often not correct or present. Studies have shown that the modality can be extracted from the image itself using visual features. Additionally, using the modality classification, the search results can be improved significantly.
- Ad-hoc image-based retrieval :
This is the classic medical retrieval task, similar to those in organized in 2005-2011. Participants will be given a set of 30 textual queries with 2-3 sample images for each query. The queries will be classified into textual, mixed and semantic, based on the methods that are expected to yield the best results.
- Case-based retrieval:
This task was first introduced in 2009. This is a more complex task, but one that we believe is closer to the clinical workflow. In this task, a case description, with patient demographics, limited symptoms and test results including imaging studies, is provided (but not the final diagnosis). The goal is to retrieve cases including images that might best suit the provided case description. Unlike the ad-hoc task, the unit of retrieval here is a case, not an image. For the purposes of this task, a "case" is a PubMed ID corresponding to the journal article. In the results submissions the article DOI should be used as several articles do not have PubMed IDs nor Article URLs.
Modality classification
The following hierarchy will be used for the modality classification, different form the classes in ImageCLEF 2011.
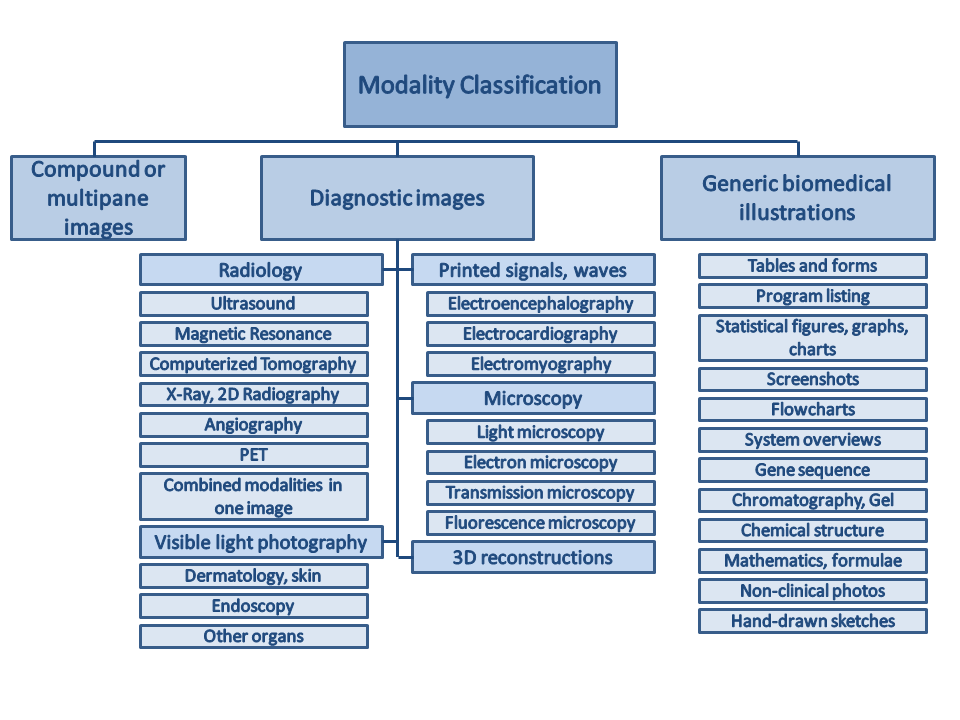
Class codes with descriptions (class codes need to be specified in run files):
([Class code] Description)
- [COMP] Compound or multipane images (1 category)
- [Dxxx] Diagnostic images:
- [DRxx] Radiology (7 categories):
- [DRUS] Ultrasound
- [DRMR] Magnetic Resonance
- [DRCT] Computerized Tomography
- [DRXR] X-Ray, 2D Radiography
- [DRAN] Angiography
- [DRPE] PET
- [DRCO] Combined modalities in one image
- [DVxx] Visible light photography (3 categories):
- [DVDM] Dermatology, skin
- [DVEN] Endoscopy
- [DVOR] Other organs
- [DSxx] Printed signals, waves (3 categories):
- [DSEE] Electroencephalography
- [DSEC] Electrocardiography
- [DSEM] Electromyography
- [DMxx] Microscopy (4 categories):
- [DMLI] Light microscopy
- [DMEL] Electron microscopy
- [DMTR] Transmission microscopy
- [DMFL] Fluorescence microscopy
- [D3DR] 3D reconstructions (1 category)
- [Gxxx] Generic biomedical illustrations (12 categories):
- [GTAB] Tables and forms
- [GPLI] Program listing
- [GFIG] Statistical figures, graphs, charts
- [GSCR] Screenshots
- [GFLO] Flowcharts
- [GSYS] System overviews
- [GGEN] Gene sequence
- [GGEL] Chromatography, Gel
- [GCHE] Chemical structure
- [GMAT] Mathematics, formulae
- [GNCP] Non-clinical photos
- [GHDR] Hand-drawn sketches
Data Download
Our database distribution includes an XML file and a compressed file containing the over 300,000 images of 75'000 articles of the biomedical open access literature.
The login/password for accessing the data is not your login/password for the registration system. In the registration system under collections, details, you can find all information on accessing the data.
Topics
We will provide 22 ad-hoc topics, divided into visual, mixed and semantic topic types.
We will also provide 26 case-based topics, where the retrieval unit is a case, not an image.
Data Submission
Image-based and case-based retrieval
Please ensure that your submissions are compliant with the trec_eval format prior to submission. We will reject any runs that do not meet the required format.
Also, please note that each group is allowed a maximum of 10 runs for image-based and case-based topics each.
The qrels will be distributed among the participants, so further runs can be evaluated for the working notes papers by the participants.
Do not hesitate to ask if you have questions regarding the trec_eval format.
At the time of submission, the following information about each run will be requested. Please let us know if you would like clarifications on how to classify your runs.
1. What was used for the retrieval: Image, text or mixed (both)
2. Was other training data used?
3. Run type: Automatic, Manual, Interactive
4. Query Language
trec_eval format
The format for submitting results is based on the trec_eval program (http://trec.nist.gov/trec_eval/) as follows:
1 1 27431 1 0.567162 OHSU_text_1
1 1 27982 2 0.441542 OHSU_text_1
.............
1 1 52112 1000 0.045022 OHSU_text_1
2 1 43458 1 0.9475 OHSU_text_1
.............
25 1 28937 995 0.01492 OHSU_text_1
where:
- The first column contains the topic number.
- The second column is always 1.
- The third column is the image identifier (IRI) without the extension jpg and without any image path (or the full article DOI for the case-based topics).
- The fourth column is the ranking for the topic (1-1000).
- The fifth column is the score assigned by the system.
- The sixth column is the identifier for the run and should be the same in the entire file.
Several key points for submitted runs are:
- The topic numbers should be consecutive and complete.
- Case-based and image-based topics have to be submitted in separate files.
- The score should be in decreasing order (i.e. the image at the top of the list should have a higher score than images at the bottom of the list).
- Up to (but not necessarily) 1000 images can be submitted for each topic.
- Each topic must have at least one image.
- Each run must be submitted in a single file. Files should be pure text files and not be zipped or otherwise compressed.
Modality classification
The format of the result submission for the modality classification subtask should be the following:
1471-2091-8-12-2 DRUS 0.9
1471-2091-8-29-7 GTAB 1
1471-2105-10-276-8 DMLI 0.4
1471-2105-10-379-3 D3DR 0.8
1471-2105-10-S1-S60-3 COMP 0.9
...
where:
- The first column contains the Image-ID (IRI). This ID does not contain the file format ending and it should not represent a file path.
- The second column is the classcode.
- The third column represents the normalized score (between 0 and 1) that your system assigned to that specific result.
You should also respect the following constraints:
- Each specified image must be part of the collection (dataset).
- An Image cannot be contained more than once.
- At least all images of the testset must be contained in runfile, however it would be nice to have the whole dataset classified.
- Only known classcodes are accepted.
Please note that each group is allowed a maximum of 10 runs.
Results
Modality classification
Runs |
Group name |
Run type |
Correctly classified in % |
Mixed |
medgift-nb-mixed-reci-14-mc.txt |
medGIFT |
Automatic |
66,2 |
medgift-orig-mixed-reci-7-mc.txt |
medGIFT |
Automatic |
64,6 |
medgift-nb-mixed-reci-7-mc.txt |
medGIFT |
Automatic |
63,6 |
Visual_Text_Hierarchy_w_Postprocessing_4_Illustration.txt |
ITI |
Automatic |
63,2 |
Visual_Text_Flat_w_Postprocessing_4_Illustration.txt |
ITI |
Automatic |
61,7 |
Visual_Text_Hierarchy.txt |
ITI |
Automatic |
60,1 |
Visual_Text_Flat.txt |
ITI |
Automatic |
59,1 |
medgift-b-mixed-reci-7-mc.txt |
medGIFT |
Automatic |
58,8 |
Image_Text_Hierarchy_Entire_set.txt |
ITI |
Automatic |
44,2 |
IPL_MODALITY_SVM_LSA_BHIST_324segs_50k_WithTextV.txt |
IPL |
Automatic |
23,8 |
Textual |
Text_only_Hierarchy.txt |
ITI |
Automatic |
41,3 |
Text_only_Flat.txt |
ITI |
Automatic |
39,4 |
Visual |
preds_Mic_Combo100Early_MAX_extended100.txt |
IBM Multimedia Analytics |
Automatic |
69,7 |
LL_fusion_nfea_20_rescale.txt |
IBM Multimedia Analytics |
Automatic |
61,8 |
preds_Mic_comboEarly_regular.txt |
IBM Multimedia Analytics |
Automatic |
57,9 |
UESTC-MKL3.txt |
UESTC |
Automatic |
57,9 |
UESTC-MKL2.txt |
UESTC |
Automatic |
56,7 |
UESTC-MKL5.txt |
UESTC |
Automatic |
56,0 |
UESTC-MKL6.txt |
UESTC |
Automatic |
56,0 |
UESTC-SIFT.txt |
UESTC |
Automatic |
52,8 |
NCFC_ORIG_2_EXTERNAL_SUBMIT.txt |
IBM Multimedia Analytics |
Automatic |
52,7 |
Visual_only_Hierarchy.txt |
ITI |
Automatic |
51,6 |
Visual_only_Flat.txt |
ITI |
Automatic |
50,3 |
gist84_01_ETFBL.txt |
ETFBL |
Automatic |
48,6 |
gist84_02_ETFBL.txt |
ETFBL |
Automatic |
48,0 |
LL_2_EXTERNAL.txt |
IBM Multimedia Analytics |
Automatic |
46,5 |
medgift-nb-visual-mnz-14-mc.txt |
medGIFT |
Automatic |
42,2 |
medgift-nb-visual-mnz-7-mc.txt |
medGIFT |
Automatic |
41,8 |
modality_visualonly.txt |
GEIAL |
Automatic |
39,5 |
medgift-orig-visual-mnz-7-mc.txt |
medGIFT |
Automatic |
38,1 |
medgift-b-visual-mnz-7-mc.txt |
medGIFT |
Automatic |
34,2 |
NCFC_500_2_EXTERNAL_SUBMIT.txt |
IBM Multimedia Analytics |
Automatic |
33,4 |
preds_Mic_comboLate_MAX_regular.txt |
IBM Multimedia Analytics |
Automatic |
27,5 |
IPL_AllFigs_MODALITY_SVM_LSA_BHIST_324segs_50k.txt |
IPL |
Automatic |
26,6 |
IPL_MODALITY_SVM_LSA_BHIST_324segs_50k.txt |
IPL |
Automatic |
26,4 |
preds_Mic_comboLate_MAX_extended100.txt |
IBM Multimedia Analytics |
Feedback or/and human assistance |
22,1 |
UNED_UV_04_CLASS_IMG_ADAPTATIVEADJUST.txt |
UNED |
Automatic |
15,7 |
UNED_UV_03_CLASS_IMG_ADJUST2MINRELEVANTS.txt |
UNED |
Automatic |
13,4 |
UNED_UV_02_CLASS_IMG_ADJUST2AVGRELEVANTS.txt |
UNED |
Automatic |
13,1 |
UNED_UV_01_CLASS_IMG_NOTADJUST.txt |
UNED |
Automatic |
11,9 |
baseline-sift-k11-mc.txt |
medGIFT |
Automatic |
11,1 |
testimagelabelres.txt |
GEIAL |
Automatic |
10,1 |
ModalityClassificaiotnSubmit.txt |
BUAA AUDR |
Manual |
3,0 |
Ad-hoc image-based retrieval
Runid |
Run type |
MAP |
GM-MAP |
bpref |
P10 |
P30 |
nlm-se |
Mixed |
0.2377 |
0.0665 |
0.2542 |
0.3682 |
0.2712 |
Merge_RankToScore_weighted |
Mixed |
0.2166 |
0.0616 |
0.2198 |
0.3682 |
0.2409 |
mixedsum(CEDD,FCTH,CLD)+1.7TFIDFmax2012 |
Mixed |
0.2111 |
0.0645 |
0.2241 |
0.3636 |
0.2242 |
mixedFCTH+1.7TFIDFsum2012 |
Mixed |
0.2085 |
0.0621 |
0.2204 |
0.3545 |
0.2152 |
medgift-ef-mixed-mnz-ib |
Mixed |
0.2005 |
0.0917 |
0.1947 |
0.3091 |
0.2 |
mixedCEDD+1.7TFIDFsum2012 |
Mixed |
0.1954 |
0.0566 |
0.2096 |
0.3455 |
0.2182 |
nlm-lc |
Mixed |
0.1941 |
0.0584 |
0.1871 |
0.2727 |
0.197 |
nlm-lc-cw-mf |
Mixed |
0.1938 |
0.0413 |
0.1924 |
0.2636 |
0.2061 |
nlm-lc-scw-mf |
Mixed |
0.1927 |
0.0395 |
0.194 |
0.2636 |
0.203 |
nlm-se-scw-mf |
Mixed |
0.1914 |
0.0206 |
0.2062 |
0.2864 |
0.2076 |
Txt_Img_Wighted_Merge |
Mixed |
0.1846 |
0.0538 |
0.2039 |
0.3091 |
0.2621 |
mixedsum(CEDD,FCTH,CLD)+TFIDFmax2012 |
Mixed |
0.1817 |
0.0574 |
0.1997 |
0.3409 |
0.2121 |
mixedFCTH+TFIDFsum2012 |
Mixed |
0.1816 |
0.0527 |
0.1912 |
0.3409 |
0.2076 |
finki/td>
| Mixed |
0.1794 |
0.049 |
0.1851 |
0.3 |
0.1894 |
finki |
Mixed |
0.1784 |
0.0487 |
0.1825 |
0.2955 |
0.1864 |
nlm-se-cw-mf |
Mixed |
0.1774 |
0.0141 |
0.1868 |
0.2909 |
0.2091 |
mixedCEDD+textsum2012 |
Mixed |
0.1682 |
0.0478 |
0.1825 |
0.3136 |
0.2061 |
FOmixedsum(CEDD,FCTH,CLD)+1.7TFIDFmax2012 |
Mixed |
0.1637 |
0.0349 |
0.1705 |
0.2773 |
0.1758 |
RFBr24+91qMixedsum(CEDD,FCTH,CLD)+1.7TFIDFmax2012 |
Mixed |
0.1589 |
0.0424 |
0.1773 |
0.3136 |
0.1985 |
medgift-ef-mixed-reci-ib |
Mixed |
0.1167 |
0.0383 |
0.1238 |
0.1864 |
0.1485 |
UNED_UV_04_TXTIMG_AUTO_LOWLEVEL_FEATURES_TWOVECTORS.txt |
Mixed |
0.004 |
0.0001 |
0.0104 |
0.0409 |
0.0258 |
UNED_UV_05_IMG_EXPANDED_FEATURES_UNIQUEVECTOR.txt |
Mixed |
0.0036 |
0.0001 |
0.0111 |
0.0455 |
0.0303 |
UNED_UV_02_IMG_AUTO_LOWLEVEL_FEATURES.txt |
Mixed |
0.0034 |
0.0001 |
0.0114 |
0.0455 |
0.0273 |
UNED_UV_08_IMG_AUTO_CONCEPTUAL_FEATURES.txt |
Mixed |
0.0033 |
0.0001 |
0.0104 |
0.0227 |
0.0197 |
IPL_AUEB_SVM_CLASS_LSA_BlockHist324Seg_50k |
Mixed |
0.0032 |
0.0002 |
0.0103 |
0.0409 |
0.0303 |
IPL_AUEB_SVM_CLASS_TEXT_LSA_BlockHist324Seg_50k |
Mixed |
0.0025 |
0.0001 |
0.0095 |
0.0318 |
0.0258 |
IPL_AUEB_CLASS_LSA_BlockColorLayout64Seg_50k |
Mixed |
0.0023 |
0.0002 |
0.0095 |
0.0318 |
0.0227 |
UNED_UV_09_TXTIMG_AUTO_CONCEPTUAL_FEATURES.txt |
Mixed |
0.0021 |
0.0001 |
0.005 |
0.0091 |
0.0061 |
IPL_AUEB_CLASS_LSA_BlockColorLayout64Seg_20k |
Mixed |
0.0019 |
0.0001 |
0.0066 |
0.0227 |
0.0197 |
UNED_UV_03_TXTIMG_AUTO_LOWLEVEL_FEATURES.txt |
Mixed |
0.0015 |
0.0001 |
0.0037 |
0.0045 |
0.0061 |
UNED_UV_07_TXTIMG_AUTO_EXPANDED_FEATURES_UNIQUEVECTOR.txt |
Mixed |
0.0015 |
0.0001 |
0.0036 |
0.0045 |
0.0061 |
UNED_UV_06_TXTIMG_AUTO_EXPANDED_FEATURES_TWOVECTORS.txt |
Mixed |
0.0013 |
0.0001 |
0.0034 |
0.0091 |
0.0045 |
UNAL |
Textual |
0.2182 |
0.082 |
0.2173 |
0.3409 |
0.2045 |
AUDR_TFIDF_CAPTION[QE2]_AND_ARTICLE |
Textual |
0.2081 |
0.0776 |
0.2134 |
0.3091 |
0.2045 |
AUDR_TFIDF_CAPTION[QE2]_AND_ARTICLE |
Textual |
0.2016 |
0.0601 |
0.2049 |
0.3045 |
0.1939 |
IPL_A1T113C335M1 |
Textual |
0.2001 |
0.0752 |
0.1944 |
0.2955 |
0.2091 |
IPL_A10T10C60M2 |
Textual |
0.1999 |
0.0714 |
0.1954 |
0.3136 |
0.2076 |
TF_IDF |
Textual |
0.1905 |
0.0531 |
0.1822 |
0.3318 |
0.2152 |
AUDR_TFIDF_CAPTION_AND_ARTICLE |
Textual |
0.1891 |
0.0508 |
0.1975 |
0.3318 |
0.1939 |
IPL_T10C60M2 |
Textual |
0.188 |
0.0694 |
0.1957 |
0.3364 |
0.2076 |
AUDR_TFIDF_CAPTION[QE2] |
Textual |
0.1877 |
0.0519 |
0.1997 |
0.3 |
0.2045 |
TF_IDF |
Textual |
0.1865 |
0.0502 |
0.1981 |
0.25 |
0.1515 |
Laberinto_MSH_PESO_2 |
Textual |
0.1859 |
0.0537 |
0.1939 |
0.3318 |
0.1894 |
IPL_TCM |
Textual |
0.1853 |
0.0755 |
0.1832 |
0.3091 |
0.2152 |
IPL_T113C335M1 |
Textual |
0.1836 |
0.0706 |
0.1868 |
0.3318 |
0.2061 |
UNAL |
Textual |
0.1832 |
0.0464 |
0.1822 |
0.2955 |
0.1939 |
TF_IDF |
Textual |
0.1819 |
0.0679 |
0.1921 |
0.2864 |
0.1909 |
TF_IDF |
Textual |
0.1814 |
0.0693 |
0.1829 |
0.2864 |
0.1894 |
UESTC-ad-tc |
Textual |
0.1769 |
0.0614 |
0.1584 |
0.3 |
0.1621 |
finki |
Textual |
0.1763 |
0.0498 |
0.1773 |
0.2909 |
0.1864 |
Laberinto_MSH_PESO_1 |
Textual |
0.1707 |
0.0512 |
0.1712 |
0.3318 |
0.1894 |
finki |
Textual |
0.1704 |
0.0472 |
0.1701 |
0.3091 |
0.1833 |
Laberinto_MMTx_MSH_PESO_2 |
Textual |
0.168 |
0.0555 |
0.1711 |
0.3227 |
0.1909 |
Terrier_CapTitAbs_BM25b0.75 |
Textual |
0.1678 |
0.0661 |
0.1782 |
0.2818 |
0.1712 |
Laberinto_MMTx_MSH_PESO_1 |
Textual |
0.1677 |
0.0554 |
0.1701 |
0.3182 |
0.1879 |
AUDR_TFIDF_CAPTION[QE2] |
Textual |
0.1673 |
0.037 |
0.1696 |
0.2955 |
0.1894 |
Laberinto_BL |
Textual |
0.1658 |
0.0477 |
0.1667 |
0.3 |
0.1939 |
AUDR_TFIDF_CAPTION |
Textual |
0.1651 |
0.0467 |
0.1743 |
0.3 |
0.2076 |
AUDR_TFIDF_CAPTION |
Textual |
0.1648 |
0.0441 |
0.1717 |
0.3318 |
0.1909 |
finki |
Textual |
0.1638 |
0.0444 |
0.1644 |
0.3 |
0.1818 |
finki |
Textual |
0.1638 |
0.0444 |
0.1644 |
0.3 |
0.1818 |
IPL_ATCM |
Textual |
0.1616 |
0.0615 |
0.1576 |
0.2773 |
0.1742 |
Laberinto_BL_MSH |
Textual |
0.1613 |
0.0462 |
0.1812 |
0.2682 |
0.1864 |
LIG_MRIM_IB_TFIDF_W_avdl_DintQ |
Textual |
0.1586 |
0.0465 |
0.1596 |
0.3455 |
0.2136 |
HES-SO-VS_CAPTIONS_LUCENE |
Textual |
0.1562 |
0.0424 |
0.167 |
0.3273 |
0.1864 |
TF_IDF |
Textual |
0.1447 |
0.0313 |
0.1445 |
0.2864 |
0.1742 |
UESTC-ad-c |
Textual |
0.1443 |
0.0352 |
0.1446 |
0.2409 |
0.1485 |
UESTC-ad-tcm |
Textual |
0.1434 |
0.051 |
0.1397 |
0.2182 |
0.153 |
LIG_MRIM_IB_FUSION_TFIDF_W_TB_C_avdl_DintQ |
Textual |
0.1432 |
0.0462 |
0.1412 |
0.2682 |
0.1955 |
LIG_MRIM_IB_FUSION_JM01_W_TB_C |
Textual |
0.1425 |
0.0476 |
0.1526 |
0.2636 |
0.1924 |
HES-SO-VS_FULLTEXT_LUCENE |
Textual |
0.1397 |
0.0436 |
0.1565 |
0.2227 |
0.1379 |
LIG_MRIM_IB_TB_PIVv2_C |
Textual |
0.1383 |
0.0405 |
0.1463 |
0.2864 |
0.1803 |
TF_IDF |
Textual |
0.1372 |
0.0466 |
0.1683 |
0.3 |
0.1818 |
Laberinto_MMTx_MSH |
Textual |
0.1361 |
0.0438 |
0.157 |
0.2091 |
0.1758 |
LIG_MRIM_IB_TFIDF_C_avdl_DintQ |
Textual |
0.1345 |
0.0402 |
0.1304 |
0.2545 |
0.1682 |
LIG_MRIM_IB_TB_JM01_C |
Textual |
0.1342 |
0.0396 |
0.142 |
0.2818 |
0.1652 |
LIG_MRIM_IB_TB_BM25_C |
Textual |
0.1165 |
0.036 |
0.1276 |
0.2 |
0.1515 |
LIG_MRIM_IB_TB_TFIDF_C_avdl |
Textual |
0.1081 |
0.0332 |
0.1052 |
0.1818 |
0.1167 |
UESTC-ad-cm |
Textual |
0.106 |
0.0206 |
0.1154 |
0.2091 |
0.1379 |
UESTC_ad_tcm_mc |
Textual |
0.101 |
0.0132 |
0.1223 |
0.2 |
0.1333 |
LIG_MRIM_IB_TB_DIR_C |
Textual |
0.0993 |
0.0281 |
0.1046 |
0.1864 |
0.1379 |
AUDR_TFIDF_CAPTION_AND_ARTICLE |
Textual |
0.0959 |
0.0164 |
0.1075 |
0.1636 |
0.1152 |
LIG_MRIM_IB_TB_TFIDF_C |
Textual |
0.09 |
0.026 |
0.0889 |
0.1409 |
0.1136 |
UESTC-ad-cm-mc |
Textual |
0.0653 |
0.0078 |
0.0846 |
0.1727 |
0.103 |
UNED_UV_01_TXT_AUTO_EN |
Textual |
0.0039 |
0.0001 |
0.0055 |
0.0091 |
0.0076 |
UNAL
|
Textual |
0.0024 |
0.0001 |
0.0113 |
0.0091 |
0.0045 |
RFBr23+91qsum(CEDD,FCTH,CLD)max2012 |
Visual |
0,0101 |
0,0004 |
0,0193 |
0,0591 |
0,0439 |
IntgeretedCombsum(CEDD,FCTH,CLD)max |
Visual |
0,0092 |
0,0005 |
0,019 |
0,05 |
0,0424 |
unal |
Visual |
0,0073 |
0,0003 |
0,0134 |
0,0636 |
0,05 |
FOmixedsum(CEDD,FCTH,CLD)max2012 |
Visual |
0,0066 |
0,0003 |
0,0141 |
0,0318 |
0,0288 |
edCEDD&FCTH&CLDmax2012 |
Visual |
0,0064 |
0,0003 |
0,0154 |
0,0409 |
0,0318 |
medgift-lf-boc-bovw-mnz-ib |
Visual |
0,0049 |
0,0003 |
0,0138 |
0,0364 |
0,0364 |
Combined_LateFusion_Fileterd_Merge |
Visual |
0,0046 |
0,0003 |
0,0107 |
0,0318 |
0,0379 |
FilterOutEDFCTHsum2012 |
Visual |
0,0042 |
0,0004 |
0,0109 |
0,0409 |
0,0364 |
finki |
Visual |
0,0041 |
0,0003 |
0,0105 |
0,0318 |
0,0364 |
EDCEDDSUMmed2012 |
Visual |
0,004 |
0,0003 |
0,0091 |
0,0364 |
0,0409 |
medgift-lf-boc-bovw-reci-ib |
Visual |
0,004 |
0,0002 |
0,0103 |
0,0227 |
0,0318 |
edFCTHsum2012 |
Visual |
0,0034 |
0,0003 |
0,01 |
0,0318 |
0,0318 |
medgift-ef-boc-bovw-mnz-ib |
Visual |
0,0033 |
0,0003 |
0,0133 |
0,0364 |
0,0333 |
UNAL |
Visual |
0,0033 |
0,0003 |
0,011 |
0,0455 |
0,0364 |
EDCEDD&FCTHmax2012 |
Visual |
0,0032 |
0,0003 |
0,0111 |
0,0227 |
0,0303 |
medgift-ef-boc-bovw-reci-ib |
Visual |
0,003 |
0,0001 |
0,01 |
0,0273 |
0,0227 |
IntgeretedCombsum(CEDD,FCTH)max |
Visual |
0,0027 |
0,0003 |
0,0099 |
0,0045 |
0,0212 |
edMPEG7CLDsum2012 |
Visual |
0,0026 |
0,0002 |
0,0058 |
0,0318 |
0,0242 |
UNAL |
Visual |
0,0024 |
0,0001 |
0,0113 |
0,0091 |
0,0045 |
medgift-lf-boc-bovw-mnz-ib |
Visual |
0,0022 |
0,0001 |
0,0062 |
0,0227 |
0,0318 |
IPL_AUEB_DataFusion_LSA_SC_CL_CSH_64seg_20k |
Visual |
0,0021 |
0,0001 |
0,0049 |
0,0273 |
0,0242 |
IPL_AUEB_DataFusion_EH_LSA_SC_CL_CSH_64seg_100k |
Visual |
0,0018 |
0,0001 |
0,0053 |
0,0364 |
0,0258 |
IPL_AUEB_DataFusion_EH_LSA_SC_CL_CSH_64seg_20k |
Visual |
0,0017 |
0,0001 |
0,0053 |
0,0227 |
0,0273 |
IPL_AUEB_DataFusion_LSA_SC_CL_CSH_64seg_100k |
Visual |
0,0017 |
0,0002 |
0,0046 |
0,0364 |
0,0212 |
baseline-sift-early-fusion-ib |
Visual |
0,0017 |
0 |
0,0058 |
0,0227 |
0,0318 |
baseline-sift-late-fusion |
Visual |
0,0016 |
0 |
0,0048 |
0,0273 |
0,0318 |
IPL_AUEB_DataFusion_EH_LSA_SC_CL_CSH_64seg_50k |
Visual |
0,0011 |
0,0001 |
0,004 |
0,0136 |
0,0136 |
IPL_AUEB_DataFusion_LSA_SC_CL_CSH_64seg_50k |
Visual |
0,0011 |
0,0001 |
0,0039 |
0,0091 |
0,0121 |
Combined_Selected_Fileterd_Merge |
Visual |
0,0009 |
0 |
0,0028 |
0,0227 |
0,0258 |
reg_cityblock |
Visual |
0,0007 |
0 |
0,0024 |
0,0227 |
0,0197 |
reg_diffusion |
Visual |
0,0007 |
0 |
0,0023 |
0,0182 |
0,0182 |
tfidf_of_pca_euclidean |
Visual |
0,0005 |
0 |
0,0011 |
0,0136 |
0,0136 |
tfidf_of_pca_cosine |
Visual |
0,0005 |
0 |
0,0013 |
0,0091 |
0,0167 |
tfidf_of_pca_correlation
|
Visual |
0,0005 |
0 |
0,0011 |
0,0136 |
0,0167 |
itml_cityblock |
Visual |
0,0001 |
0 |
0,0014 |
0,0045 |
0,003 |
itml_diffusion |
Visual |
0,0001 |
0 |
0,0015 |
0,0045 |
0,003 |
Case-based retrieval
Runid |
Run type |
MAP |
GM-MAP |
bpref |
P10 |
P30 |
medgift-ef-mixed-mnz-cb |
Mixed |
0,1017 |
0,0175 |
0,0857 |
0,1115 |
0,0679 |
medgift-ef-mixed-reci-cb |
Mixed |
0,0514 |
0,009 |
0,0395 |
0,0654 |
0,0564 |
HES-SO-VS_FULLTEXT_LUCENE |
Textual |
0,169 |
0,0374 |
0,1499 |
0,1885 |
0,109 |
LIG_MRIM_CB_FUSION_DIR_W_TA_TB_C |
Textual |
0,1508 |
0,0322 |
0,1279 |
0,1538 |
0,1167 |
LIG_MRIM_CB_FUSION_JM07_W_TA_TB_C |
Textual |
0,1384 |
0,0288 |
0,11 |
0,1615 |
0,1141 |
UESTC_case_f |
Textual |
0,1288 |
0,025 |
0,1092 |
0,1231 |
0,0821 |
UESTC-case-fm |
Textual |
0,1269 |
0,0257 |
0,1117 |
0,1231 |
0,0821 |
LIG_MRIM_CB_TFIDF_W_DintQ |
Textual |
0,1036 |
0,0167 |
0,077 |
0,0846 |
0,0705 |
nlm-lc-total-sum |
Textual |
0,1035 |
0,0137 |
0,1053 |
0,1 |
0,0628 |
nlm-lc-total-max |
Textual |
0,1027 |
0,0125 |
0,1055 |
0,0923 |
0,0538 |
nlm-se-sum |
Textual |
0,0929 |
0,013 |
0,0738 |
0,0769 |
0,0667 |
nlm-se-max |
Textual |
0,0914 |
0,0128 |
0,0736 |
0,0769 |
0,0667 |
nlm-lc-sum |
Textual |
0,0909 |
0,0133 |
0,0933 |
0,1231 |
0,0654 |
LIG_MRIM_CB_TA_TB_JM07_C |
Textual |
0,0908 |
0,0156 |
0,0799 |
0,1308 |
0,0744 |
LIG_MRIM_CB_TA_TB_BM25_C |
Textual |
0,0895 |
0,0143 |
0,0864 |
0,1231 |
0,0654 |
LIG_MRIM_CB_TA_TB_DIR_C |
Textual |
0,0893 |
0,0137 |
0,0804 |
0,1192 |
0,0692 |
LIG_MRIM_CB_TA_TB_PIVv2_C |
Textual |
0,0865 |
0,0158 |
0,0727 |
0,1192 |
0,0795 |
nlm-lc-max |
Textual |
0,084 |
0,0109 |
0,0886 |
0,0923 |
0,0603 |
LIG_MRIM_CB_TA_TFIDF_C_DintQ |
Textual |
0,0789 |
0,014 |
0,0672 |
0,0923 |
0,0692 |
nlm-se-frames-sum |
Textual |
0,0771 |
0,0052 |
0,0693 |
0,0692 |
0,0526 |
HES-SO-VS_CAPTIONS_LUCENE |
Textual |
0,0696 |
0,0028 |
0,0762 |
0,0962 |
0,0615 |
LIG_MRIM_CB_TA_TB_TFIDF_C_avdl |
Textual |
0,0692 |
0,0127 |
0,0688 |
0,0769 |
0,0692 |
nlm-se-frames-max |
Textual |
0,0672 |
0,0031 |
0,0574 |
0,0538 |
0,05 |
LIG_MRIM_CB_TA_TB_TFIDF_C |
Textual |
0,0646 |
0,0114 |
0,0624 |
0,0692 |
0,0641 |
ibm-case-based |
Textual |
0,0484 |
0,0023 |
0,0439 |
0,0577 |
0,0449 |
R1_MIRACL |
Textual |
0,0421 |
0,005 |
0,026 |
0,0538 |
0,0462 |
R4_MIRACL |
Textual |
0,0196 |
0,0008 |
0,0165 |
0,0308 |
0,0282 |
R3_MIRACL |
Textual |
0,012 |
0,0004 |
0,0087 |
0,0192 |
0,0218 |
R6_MIRACL |
Textual |
0,0111 |
0,0004 |
0,0074 |
0,0192 |
0,0128 |
R5_MIRACL |
Textual |
0,0024 |
0 |
0,0022 |
0,0038 |
0,0013 |
R2_MIRACL |
Textual |
0 |
0 |
0,0002 |
0 |
0 |
medgift-lf-boc-bovw-reci-IMAGES-cb |
Visual |
0,0366 |
0,0014 |
0,0347 |
0,0269 |
0,0141 |
medgift-lf-boc-bovw-mnz-IMAGES-cb |
Visual |
0,0302 |
0,001 |
0,0293 |
0,0231 |
0,009 |
baseline-sift-early-fusion-cb |
Visual |
0,0016 |
0 |
0,0032 |
0,0038 |
0,0013 |
baseline_sift_late_fusion_cb |
Visual |
0,0008 |
0 |
0 |
0,0038 |
0,0013 |
medgift-ef-boc-bovw-reci-IMAGES-cb |
Visual |
0,0008 |
0,0001 |
0,0007 |
0 |
0,0013 |
medgift-ef-boc-bovw-mnz-IMAGES-cb |
Visual |
0,0007 |
0 |
0 |
0 |
0,0013 |
Organizers
- Henning Müller, HES-SO, Switzerland
- Jayashree Kalpathy-Cramer, Harvard University, USA
- Dina Demner-Fushman, National Library of Medicine, USA
- Sameer Antani, National Library of Medicine, USA
- Alba García Seco de Herrera, HES-SO, Switzerland